Digital Tools for Better Lending Capital Allocation
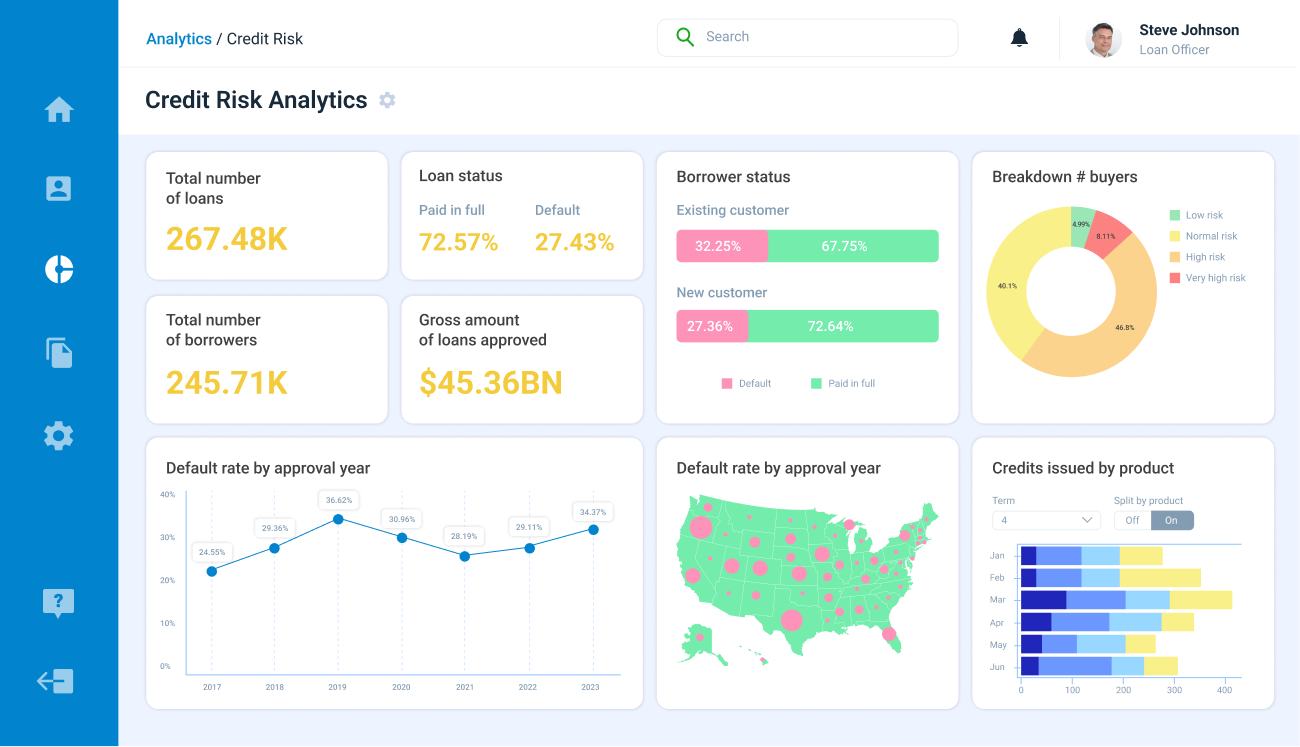
Editor’s note: Dimitry Senko, Lending IT Consultant at ScienceSoft, explains the shortcomings of traditional modeling and forecasting techs and suggests better tools for efficient lending capital allocation. Dimitry’s primary focus is on tactical allocations in the loan underwriting and portfolio management areas. The interview is led by Stacy Dubovik, a financial technology researcher.
Capital Misallocation in Lending: Where the Gaps Stem From
SD (Stacy Dubovik): In your experience, what is the biggest hindrance to efficient capital allocation for lenders?
DS (Dimitry Senko): I’d say that the need to adjust plans and address versatile risks agilely is the primary challenge. Since any lender’s core operational activity — issuing loans — is a form of capital allocation itself, lenders make micro-scale allocation decisions during underwriting on a daily basis. These tactical capital deployments directly impact portfolio-level and organization-wide returns and liquidity. To maximize overall yield while securing financial health, you need to dynamically right-size your loan portfolios and strategic projections. However, a recent research by Morgan Stanley revealed that 98% of companies lack operational and analytical agility to reallocate continuously, which results in their suboptimal financial performance.
At the tactical level, the biggest obstacles are systemic credit and liquidity risks. To mitigate them, you need two things. First, you should determine who, how much, and at what price you want to lend money to in order to maximize the risk-weighted returns on your limited funds. Second, you should accurately plan your portfolio-level cash flows and capital recycling to maintain healthy liquidity levels. Both tasks are complex due to the high degree of uncertainty.
Close dependence on macroeconomic conditions is another hurdle. Even minor economic downturns commonly lead to a spiky rise in defaults, forcing lenders to reallocate reserves to provisions for loan losses on the fly. To compensate for potential capital impairments, you need to factor in anticipated borrower conduct during market shifts when pricing each particular loan. From what I observe, such risks are rarely sufficiently captured. And even if they are, turbulent interest rates and borrowers’ disordered behaviors amidst crisis might still compromise initially reasonable decisions.
For bank lenders, the task is further complicated by stringent capital reserve requirements. Following Basel III, the Fed mandates US banks to maintain capital buffers for liquidity and solvency, and its requirements are changing frequently. To stay compliant, banks must allocate with reserves in mind, monitor the Fed’s revisions, and have a go-to funding plan to bridge liquidity shortfalls. The 2023 collapse of Silicon Valley Bank is a prime example of where dramatic regulatory moves, lending risk mismanagement, and unfavorable market conditions may lead.
I recently came across research by EY where over 50% of companies named delayed data access and poor analytics as their biggest barriers to optimal capital allocation. Indeed, a lender’s legacy analytics toolkit is a serious setback for effective decisioning. You need intelligent data processing, financial modeling, and forecasting engines to keep up with the dynamic risk landscape, but this is exactly what old-school tools are missing with their limited data integration options and rule-based reasoning.
SD: But how do you even detect capital misallocation if the company is showing consistent growth on the surface? SVB’s case proves you could be on the rise one day and collapse the next.
DS: Unfortunately, misallocation can still be a problem for the thriving company, and it’s easy to overlook gaps when you’re on the up. My previous experience working for a large bank lender shows that positive top-line numbers can sometimes mask serious inefficiencies in tactical capital deployment. Periods of tight liquidity make misallocations apparent, but it’s often too late for painless makeshifts. Again, many of these issues stem from data opacity and weak analytics mechanisms.
For example, poor analytics can amplify concentration risks by failing to account for correlations and underlying vulnerabilities within a portfolio. A common pitfall: you might gradually skew your loan portfolio toward a particular product — say, commercial real estate loans — because it historically performed well. On the surface, this looks like a sound strategy, especially since your analytics prove these loans are contributing to growth. However, if allocations are not sufficiently diversified, you become overly exposed to downturns in that specific market.
Another source of failure is inconsistent loan and funding strategies. Say you’ve been mainly committing tactical capital to long-term fixed-rate loans while your funding sources rely on short-term borrowing. Even as your loan book grows, mismatched maturity and interest rate risks can squeeze your margins if market conditions shift (e.g., if funding costs increase). Poor analytics might obscure these imbalances and their impact on liquidity in changing settings. And without dynamic market predictions, you will miss early signs of rising funding costs.
However, I believe the biggest hidden inefficiency comes from underwriting. With rigid credit risk assessment models, suboptimal capital allocations may remain unnoticed for years. Static models calculate credit scores based on a limited range of borrower data and fail to capture nuanced risks. In practice, it means you might overallocate to borrowers who are qualified low-risk but have hidden vulnerabilities. At the same time, you might underallocate to mid-risk borrowers who, upon a deeper assessment, could generate way better risk-adjusted returns. I overviewed this specific issue in my recent guide to digital credit underwriting strategies.
What Analytics Components You Need for Efficient Capital Allocation in 2025
SD: Let’s focus on the tactical level. You mentioned that an average lender’s legacy analytics toolkit doesn’t cut it anymore. What new technologies do lenders need to improve loan and portfolio allocations?
DS: Modeling and forecasting tools are the primary candidates for upgrading, as they are critical to every capital decision, from issuing individual loans to strategic portfolio planning. Here, traditional statistical models may still work for one-time projections like evaluating portfolio diversification or loan securitization potential. However, dependence on pre-processed data, static assumptions, and linear input relationships makes them unfeasible for dynamic predictions across disparate variables.
AI-powered predictive analytics can effectively address these shortcomings. Such tools can rapidly process large-volume and diverse datasets relevant to capital allocation decisions. Machine learning (ML) forecasting models can identify nuanced relationships within these data points and accurately predict their impact on allocation risks and profitability.
For example, intelligent algorithms can capture the correlations between borrower spending patterns and macroeconomic indicators and suggest how they may affect default probability. Similarly, they can analyze real-time exposures across various loan types, geographies, and borrower segments and pinpoint high-risk portfolio clusters that may compromise future yield and liquidity. Bringing predictive analytics in underwriting helps lenders increase loan approval rates by 15–50% while reducing credit losses by 20–40% — a huge gain from the RAROC perspective. At the portfolio level, you may expect, on average, 20–40% more accurate health and performance predictions with a comparable uplift in allocation efficiency.
The powerful diagnostic capabilities of machine learning models can reinforce root cause analysis and capital deployment optimization. For example, smart algorithms can dissect loan repayment factors and pinpoint whether rising delinquencies across particular loan types stem from economic downturns, sector-specific issues, or changes in borrower behaviors. These insights let lenders take precise corrective actions: downsize the allocation to underperforming portfolio segments, adjust loan terms, or modify certain underwriting criteria for sharper risk scoring.
Unlike traditional tools, intelligent analytics can effectively process unstructured and semi-structured data. This gives you an opportunity to integrate new, previously unused data feeds in loan and portfolio risk projections. High-quality alternative data contributes to the precision of capital-related forecasts: for example, lenders who employed borrower transactional histories for risk scoring report up to a 10% increase in risk prediction accuracy.
Not all analytics tools offer dynamic tracking and sensing of lending metrics, but you need this capability for enhanced capital planning and risk management. My lending clients who launched dynamic control tools say it helped them allocate more profitably and shift from inefficient reactive reallocations to proactive capital rescue. Your solution must continuously capture changing borrower, portfolio, liquidity, and market variables, calculate the necessary capital-related KPIs, and automatically adjust yield and exposure projections. Automated alerts on critical values nearing thresholds will help your teams quickly spot escalating risks and take timely actions. Look for a tool that supports dynamic Monte Carlo simulations to better plan for uncertain market and economic events.
Also, prioritize intuitive dashboards with drill-down and drill-up capabilities to make it easier for your teams to navigate multi-level capital metrics. When it comes to visualization, make sure your analytics solution features time-series charts, waterfall charts, interactive scatter plots, and other specialized components that help represent analytical results in an insightful way.
Sample dashboards of credit risk analytics by ScienceSoft. Follow the link to explore what data and KPIs you can control with a comprehensive lending analytics solution.
SD: These days, many talk about the power of prescriptive AI and intelligent decision-making, but you haven’t mentioned this capability. Does that mean it doesn’t have much value for tactical capital allocation?
DS: I’m not downplaying the general potential of prescriptive tools. But at this point, AI just isn’t smart enough to make the optimal decisions and deploy tactical capital autonomously. Due to their predefined and restrained training datasets, modern AI models struggle to effectively address unique cases that are not rare in real-life lending settings. For example, AI might misinterpret unique macro scenarios where historical data offers little guidance, like sudden geopolitical crises or pandemics, and suggest portfolio resizing based on irrelevant patterns. The rigidity might also cause AI to deny loan restructuring for a promising startup from an emerging domain, while a human loan officer could find innovative ways to adjust credit terms to mutual advantage.
Plus, neither humans nor machines are immune to making wrong decisions. But if AI goes haywire and misallocates in high-stake cases, who’s to blame? Many lending leaders I talked to named vague responsibility and accountability for AI-induced losses among the top reasons for avoiding prescriptive AI in capital management tasks.
You can still use prescriptive AI for granular suggestions. For example, intelligent assistants could quickly advise your operating teams on loan adjustment options, potential portfolio expansions, or ways to bridge liquidity gaps. But I always warn my lending clients against treating smart suggestions as an ultimate source of truth for big decisions.
Best Practices for Implementing New Capital Analytics Solutions
SD: So, do lenders have to retire their old capital management tools completely to roll out intelligent analytics? Or is there a way to implement some smart tools alongside the legacy systems?
DS: It depends on the nature and maturity of the lender’s software. At ScienceSoft, we, by default, try not to discard heritage loan and portfolio solutions but to augment them with modern analytical components. This is the quickest, cheapest, and least disruptive way to enhance the capital allocation tool stack. From my experience, a custom AI component for credit risk assessment or loan portfolio forecasting can be engineered within the budget of $100,000–$250,000. Lending AI platforms like Zest AI offer cheaper, ready-made frameworks for building predictive ML models, but they come with tradeoffs in accuracy and available integrations.
At the same time, I can’t be too optimistic: legacy lending systems are often too rigid to accommodate modern add-ons. You may need to partially revamp particular components to make your system suited for advanced analytics. Such workarounds may sometimes cost more than building new software from scratch. In this case, I recommend that my clients incrementally replace their existing tools with modern software. Developing a custom analytics solution for product-level capital allocation (e.g., a credit risk analytics system) may require a budget of $200,000–$600,000 with an average payback period of 9 months. As an alternative, you can implement a fitting commercial software product and customize it to your needs. Software products are cheaper initially, but in three years, they will accumulate about the same TCO as custom solutions, and in the long term, custom becomes cheaper thanks to no recurring license fees.
Some scenarios leave no choice. For example, you won’t be able to avoid implementing new software if you manage tactical allocations in spreadsheets or simplistic tools. Such solutions do not support — and cannot be upgraded to support — the integration and automated processing of data from multiple sources, which is a fundamental requirement for dynamic analytics. On the flip side, basic tools can be retired at no cost; you’ll only need to invest in data migration.
SD: Where should a lender start to secure a high ROI for its tactical analytics project?
DS: Begin by clearly defining the strategic objectives of your initiative, whether it’s reducing loan risks, increasing the profit margin on each borrower, driving portfolio returns, or stabilizing liquidity. This will help you determine the primary area of improvement and focus on the analytics capabilities that address the biggest capital allocation pains. If you pursue a complete transformation, underwriting is a sweet spot to start with. As this area is the major driver for both margin and risks, improving it commonly leads to the highest capital efficiency gains.
Involve stakeholders from credit, finance, and risk management teams to define requirements for the analytics solution. Their early insights will ensure you build the solution that meets their exact operational needs and won’t end up underutilized. The input of subject matter experts is also precious for the accurate design and calibration of specialized analytical models like credit risk assessment algorithms for a particular loan type or capital adequacy assessment models for region-specific sub-portfolios.
Eliminating data silos should be your top priority. From my experience, it’s not uncommon for lenders to use separate software and databases for underwriting, debt collection, portfolio management, and general finance. If some systems fail to integrate and share data, critical datasets may be isolated. In contrast, your analytics solution will need immediate access to capital-relevant data to operate effectively and deliver accurate insights. Implementing an automated data consolidation platform or a data warehouse may be a necessary step to enabling effective analytics.
Any gaps and flaws in training datasets can limit the accuracy of intelligent analytical models, so it’s important to build a high-quality data framework first. If you set out for AI-supported analytics, evaluate the multi-dimensional data you’re planning to use for machine learning model training. Assess data quality, consistency, and availability across your corporate systems. If you want to leverage third-party data feeds, say, for credit risk or market analytics, pick reliable sources and plan data acquisition proactively — enabling integrations with external systems might take up to six months.
If you need advice on the optimal capital allocation toolkit or digital transformation strategy for your case, feel free to contact me or other consultants at ScienceSoft.